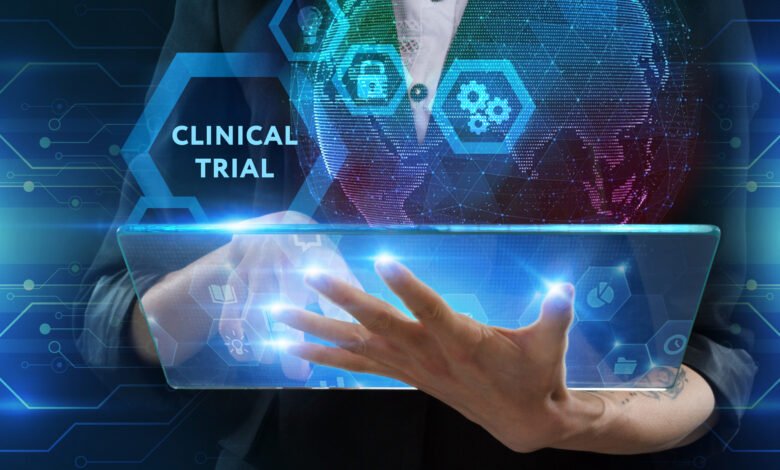
The future of Conditional Random Field (CRF) design holds great promise as advancements in technology continue to shape the landscape of machine learning and natural language processing. In this era of rapid innovation, staying abreast of the latest tech trends is crucial for researchers and practitioners in the field. Let’s delve into the exciting developments and emerging technologies that are set to revolutionize CRF design in the near future. In this article, we will explore these trends and their implications for the future of technology.
1. Integration of Machine Learning and CRF Design
One of the most significant trends in CRF design is the integration of machine learning algorithms. Machine learning techniques, such as deep learning and neural networks, are being combined with CRF design to enhance the efficiency and effectiveness of reinforcement learning systems. By leveraging the power of machine learning, CRF design can adapt and learn from data in real-time, leading to more intelligent and autonomous systems.
The integration of Machine Learning (ML) and Conditional Random Fields (CRF) design has revolutionized the field of natural language processing and sequence labeling tasks. Machine Learning algorithms, particularly deep learning models like neural networks, have shown remarkable success in various tasks such as image recognition, speech recognition, and text classification. However, when it comes to sequential data like text, traditional ML models struggle to capture the dependencies between neighboring tokens effectively. This is where CRFs come into play.
Conditional Random Fields are a type of probabilistic graphical model that is well-suited for sequential data labeling tasks. By combining the power of ML algorithms for feature extraction and CRFs for modeling sequential dependencies, researchers have been able to achieve state-of-the-art results in tasks like named entity recognition, part-of-speech tagging, and information extraction. The integration of ML and CRF design allows for more accurate and context-aware predictions, leading to improved performance in various natural language processing applications.
Overall, the synergy between Machine Learning and CRF design has paved the way for more sophisticated and effective models in sequence labeling tasks, pushing the boundaries of what is possible in the realm of natural language processing.
2. Explainable AI in CRF Design
Explainable Artificial Intelligence (AI) is gaining traction in the field of CRF design. As CRF systems become more complex and sophisticated, the ability to interpret and explain their decisions becomes crucial. Explainable AI techniques allow researchers and developers to understand how CRF systems arrive at their decisions, enabling greater transparency and trust in these systems.
3. Quantum Computing and CRF Design
The advent of quantum computing is set to revolutionize CRF design. Quantum computers have the potential to solve complex optimization problems at a speed and scale that is currently unattainable with classical computers. By harnessing the power of quantum computing, CRF design can achieve breakthroughs in optimization and decision-making, leading to more efficient and robust systems.
4. Edge Computing for Real-Time CRF Applications
Edge computing is another trend that is shaping the future of CRF design. By processing data closer to the source, edge computing enables real-time decision-making and analysis, making it ideal for CRF applications that require low latency and high reliability. The integration of edge computing with CRF design opens up new possibilities for autonomous systems in dynamic and unpredictable environments.
5. Interdisciplinary Collaboration in CRF Design
Collaboration across disciplines is becoming increasingly important in CRF design. As CRF systems become more complex and multifaceted, experts from diverse fields, such as computer science, engineering, psychology, and neuroscience, are coming together to tackle the challenges of designing intelligent and adaptive systems. Interdisciplinary collaboration fosters innovation and creativity, leading to groundbreaking advancements in CRF design.
6. Ethical Considerations in CRF Design
Ethical considerations are becoming a central focus in CRF design. As CRF systems become more autonomous and pervasive in society, questions of ethics, bias, and accountability arise. It is essential for researchers and developers to address these ethical considerations proactively and ensure that CRF systems are designed and deployed responsibly, with the well-being of society in mind.
Conclusion
The integration of Machine Learning and Conditional Random Fields (CRF) design offers a powerful approach for structured prediction tasks. By combining the strengths of ML algorithms with the sequential modeling capabilities of CRFs, more accurate and robust predictions can be achieved. This synergy enhances the performance of various applications such as named entity recognition, part-of-speech tagging, and information extraction. For more insights on technology and innovation, visit https://techhbs.com.